Part of ECMWF's 50th anniversary celebrations
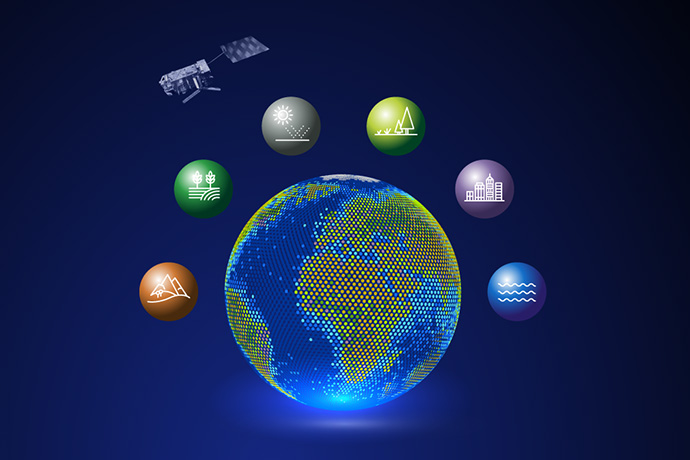
Overview
Recent advances in land surface and more general Earth system models lead to higher resolution and increased fidelity in the representation of local processes. To allow for the correct representation of these processes, it is necessary to seek increased quality, complexity, appropriateness and availability of ancillary fields that are used to constrain land surface models and their related parameters. With a wide spectrum of ancillary data including topography, physiographic fields, vegetation structures and indices, and soil related hydrological parameters, the data providers range between satellite agencies to in situ data investigators. This makes it difficult to define and harmonise the data requirements. To develop appropriate data for future modelling needs, it is therefore essential for modellers and data providers to deepen their mutual understanding of the challenges involved in the generation of the data and evolving modelling requirements. This workshop is an opportunity to bridge the gap between these different communities.
Additionally, machine learning is also changing the landscape as it is opening new possibilities to improve these datasets via a more automated extraction of information from observations, to improve links between the model simulations and the end users, and to improve pure machine learning forecast models and parameter optimisation that will heavily depend on the provision of good background information.
The workshop will summarise the current state of the art, pros and cons of existing data, and advise on data requirements. This would result in outlining potential changes in the workflow and fields that are generated and stimulate the production of improved datasets for the next generation of land surface models.
Expected outcomes
The workshop is expected to enhance the communication and coordination between data providers and weather and climate centres, leading to a better understanding of:
- the requirements of future land-surface models for the accurate representation of processes at high resolution and the need for future observations and missions to support these developments.
- methods to bridge the gap across spatial and temporal scales and to represent spatial heterogeneity.
- the representation of data uncertainties and limits of products.
- how machine learning could help for field generation, gap filling and parameters optimisation.
- the processes for quality assurance, standardisation, documentation and curation of data and software.
Attendance information
If you would like to attend this workshop, please complete the registration form before 13 December 2024. If you are interested in giving a presentation/poster at the workshop, please also submit an abstract submission form before this date.